
Technology of the Future: Machine Learning
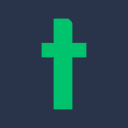
Machine learning is a branch of artificial intelligence that enables computers to learn by extracting meaning from data. In this process, algorithms and models are used to analyze data. It makes predictions or classifications based on this data. For example, it has the ability to classify an object in an image based on its color, shape, or other features. Similarly, it can categorize incoming emails into groups such as social, advertising, or priority. These processes are carried out through machine learning algorithms.
How is Machine Learning Applied?
Machine learning applications are implemented through various methods. The process starts by collecting the type of data required for the model. For instance, tumor detection applications gather radiology images, while facial recognition applications collect facial images. Necessary features are defined to make sense of unstructured data. After the data is prepared, it is divided into training and test groups. Based on the application’s needs, the appropriate machine learning algorithm is selected. Once the training process is completed, the model is evaluated with test data, and its performance is measured.
Differences Between Data Science and Machine Learning
Machine learning is a subfield of data science and differentiates itself through its automatic learning and prediction abilities. Data science encompasses a wide range of processes, such as data collection, analysis, and processing. Machine learning, on the other hand, focuses specifically on automatic learning processes from data. Data science aims to derive meaningful insights from data using visualization and statistical analysis. Machine learning, however, creates models from the data and uses these models to make predictions.
Types of Machine Learning
Machine learning is mainly divided into three categories: supervised learning, unsupervised learning, reinforcement learning, and deep learning. Among these, deep learning and supervised learning methods are commonly preferred. Supervised learning allows a model to learn the relationship between inputs and the correct outputs from the training data set. Deep learning, on the other hand, excels at processing large and complex data by using multi-layered neural networks. As the amount of data increases, the model's accuracy also improves.
The Role of Deep Learning in Machine Learning
Deep learning, also known as artificial neural networks, differs from other algorithms by automatically identifying complex patterns in data and minimizing the need for feature engineering. An example of this is chatbots used in customer support services by banks, which are powered by neural networks. These bots, through natural language processing techniques, can interpret users' questions and provide accurate responses.
Applications of Machine Learning in Daily Life
Today, machine learning techniques are utilized in many areas. For instance, in healthcare, it aids in the early diagnosis of diseases like cancer and diabetes through image processing techniques. In finance and banking, it is used in areas like fraud detection. In the legal field, it helps classify case files using natural language processing. It is also present in fields such as education, transportation, and agriculture.
The Future of Machine Learning and Ethical Issues
The rapid integration of machine learning into our lives is leading to automation and changes in the demand for human labor. Ethical concerns are becoming increasingly significant in relation to these developments. Issues such as personal data security and privacy are subject to debate. Machine learning will continue to play a crucial role in our world not only today but also in the future. However, we must not forget our responsibilities to ensure that these opportunities serve society in the best way possible. This responsibility rests not only on engineers but on all of us.